I used the new family of MIRAI (Multiple Index Regression for AI) and made model equations.
The original data is a new test method proposal from the National Institute of Health Sciences Japan, ‘Alternative method for skin irritation testing using a human skin model (3D skin model EPISKIN)’.
I don’t understand what’s in it.
I am currently trying to start an alternative animal testing method in the pirika research group with my students to see if it is possible to predict Hansen’s solubility parameters and other parameters.
This is what I found when I was searching the internet.
These alternative testing methods and QSARs with machine learning will become very important as Europe moves towards a ban on animal testing.
Hansen Solubility Parameters (HSP) are very important as an identifier for machine learning.
After all, there is no poison or medicine unless it enters the body through the skin.
Other topological information such as logKow (octanol-water partition ratio), logS (solubility in water), logBCF (bioaccumulation, not yet included in HSPiP) and RDKit can also be used.
Mixtures cannot be calculated, but the Smiles structural formulae and Dominant Median values of the compounds used in this study are given below, so if you think you have what it takes, please try your hand at creating a model equation.
I am not familiar with Dominant Median, but it is said to be 2.3 or higher for Irritant, 1.5 to 2.3 for Mild irritant and 1.5 or lower for Non-Irritant according to the GHS classification.
In other words, it is sufficient if the Dominant Median can be predicted from the structure of the molecule only.
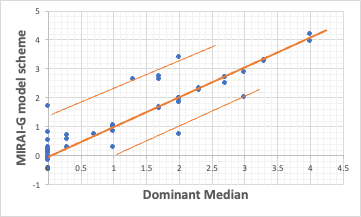
The analysis tool developed by Pirika allows the user to choose between four different methods for taking errors when constructing the model equation.
In this case, the analysis with MAE produced the results shown above, with 40 of the 49 data points riding almost neatly on a Y=X straight line.
The results also show that there are five compounds that are polarised upwards and three compounds that are polarised downwards.
In other words, modelling with the MAE of MIRAI-G shows that 40 compounds are well described by the identifiers chosen this time, but two more identifiers are missing.
We can think about what characteristics the compounds that are polarised upwards and downwards have.
This is a big difference from the neural network method, where you have a black box inside and you don’t know why, but everything is on average on the line.